POGO
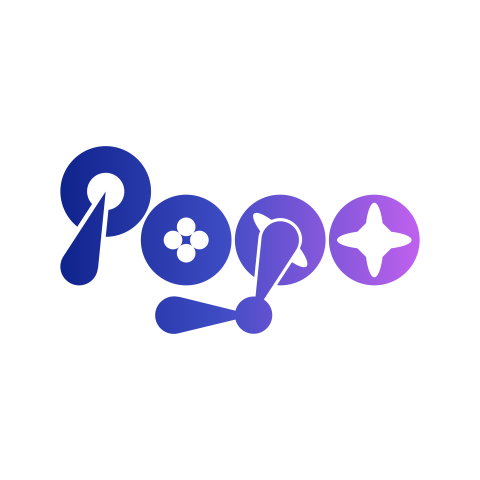
POGO
Technological advancement within the last half century can largely be attributed to the downscaling of transistors: Smaller transistors fit onto chips in greater numbers, directly increasing the amount of compute power we can achieve. ASML defines the frontier of this pursuit as the world's sole provider of nanometer-scale fabrication machines.
ASML's proprietary process etches chips with high-energy ultraviolet light, which is generated by the evaporation of tin droplets. This highly sophisticated machinery operates on a multitude of settings, and costs hundreds of thousands of dollars just to run, even among ASML internal teams. Motivated by immense data complexity and steep data cost, our capstone team was tasked with generalizing the relationship between tin droplet geometry and EUV yield.
POGO (Parameter Operated Geometric Optimizer) is a generative machine learning model trained to infer droplet geometries given a set of input parameters. It is capable of exploring untested machine settings with high confidence and is adaptable to new datasets. It is also able to approximate parameters given images of tin droplets.
As we hand off this project, it is our sincere hope that ASML will utilize POGO-informed machine inputs in EUV optimization, that we have aided them in refining their preeminent technology.